Research & Development
With over 50+ years of combined industry and academic experience in AI and ML operations, Arthur is proud to adopt a research-led approach to product development. Our experimental approach and expert researchers drive exclusive capabilities in LLMs, computer vision, bias mitigation, and other critical areas.
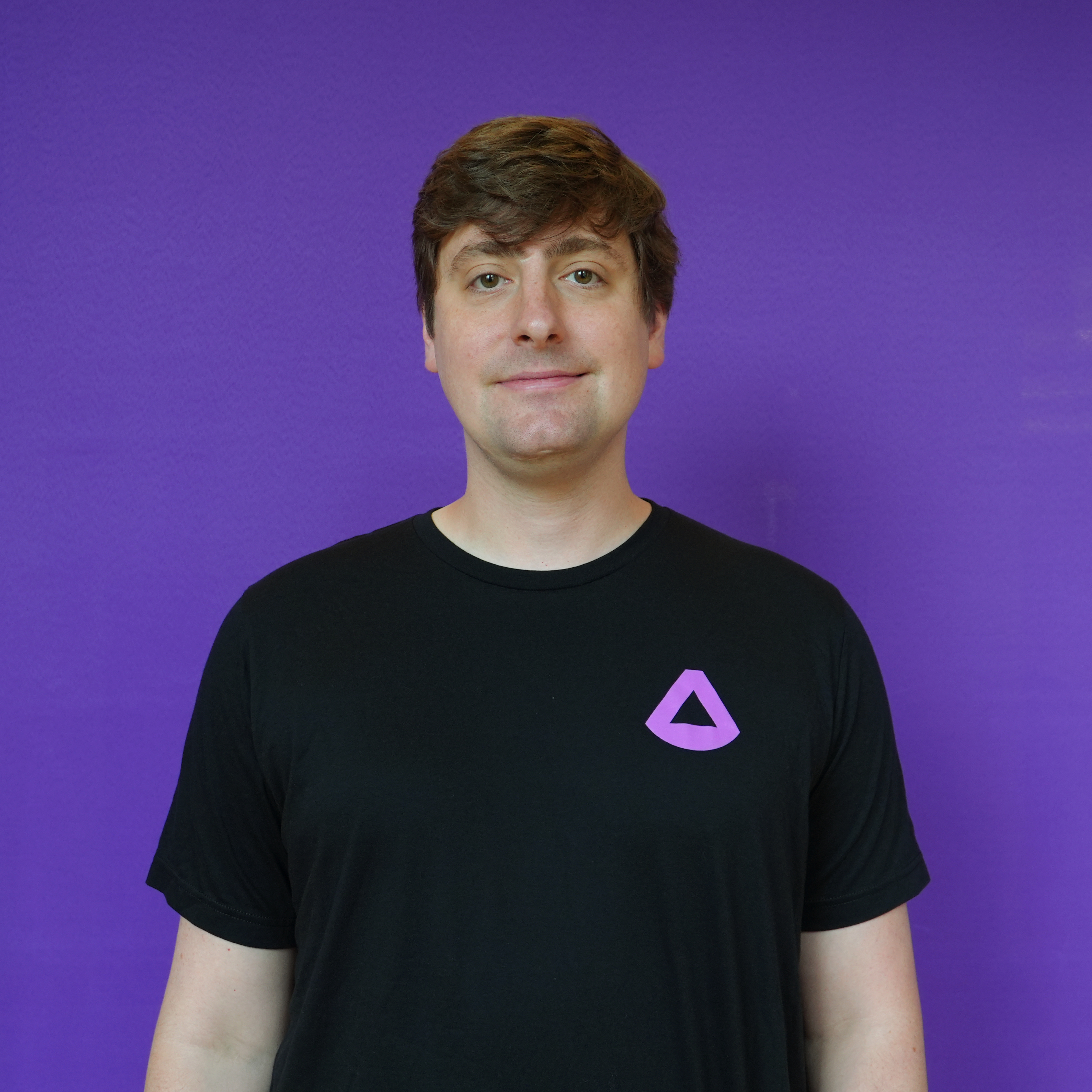
John Dickerson
Chief Scientist
John serves as Co-Founder of and Chief Scientist to Arthur. He is also an Assistant Professor in the Department of Computer Science at the University of Maryland. He specializes in solving problems at the intersection of economics and artificial intelligence using techniques from machine learning, stochastic optimization, and computational social choice. He has worked extensively in the design and fielding of markets in healthcare and in advertising. John brings to Arthur a unique blend of academic pursuit and industry problem solving. He holds a PhD in Computer Science from Carnegie Mellon University.
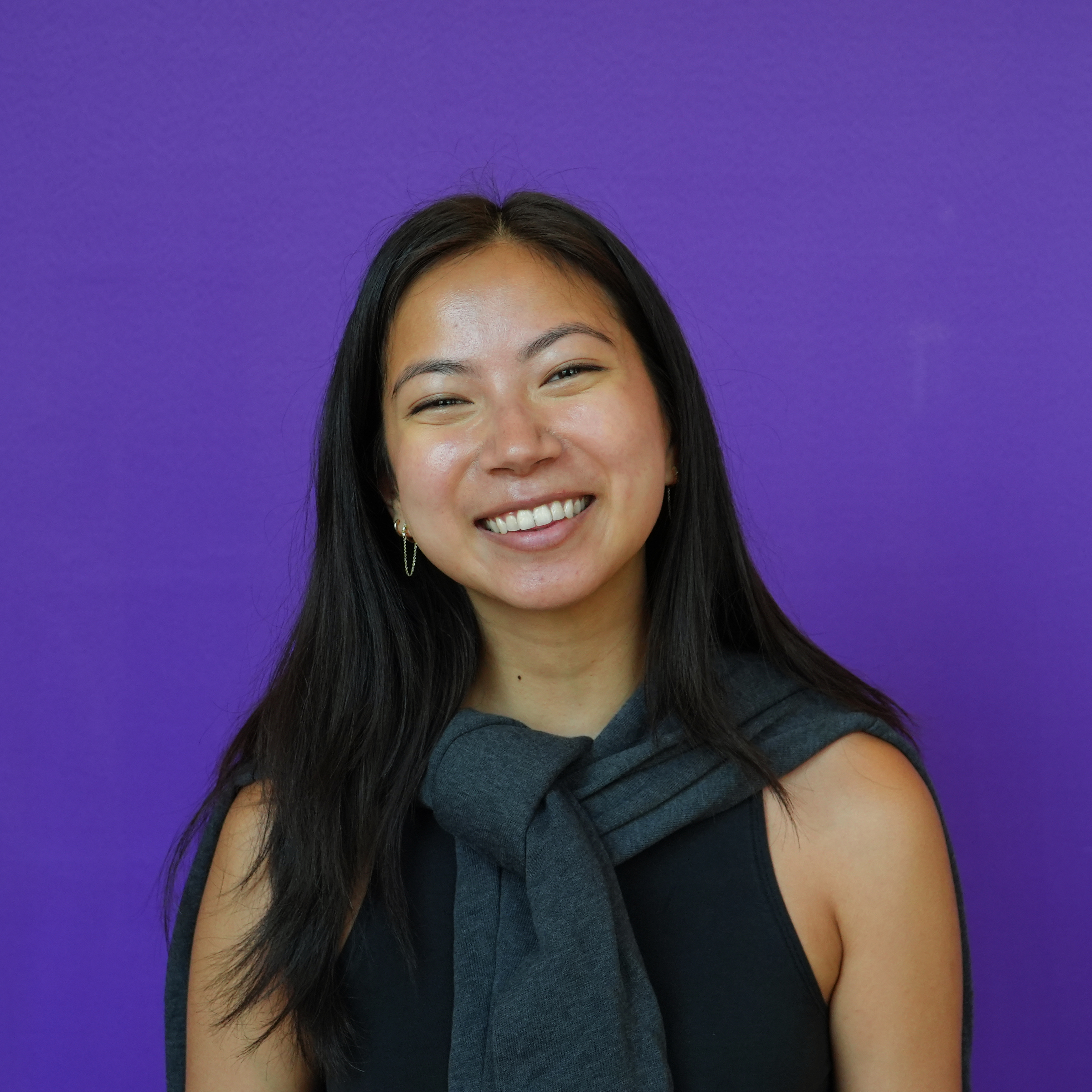
Teresa Datta
Machine Learning Engineer
Teresa is a researcher at Arthur interested in transparency and social impact of algorithmic systems from a human-centered lens. She is interested in use-case evaluations of tools for AI transparency and context-based mechanisms for accountability. Previously, she worked on XAI and HCI projects while completing her M.S. in Data Science at Harvard University.
.jpg)
Max Cembalest
Machine Learning Engineer
Max is a researcher at Arthur focused on simplifying and explaining machine learning models. Previously, he received an M.S. in Data Science from Harvard University, where he concentrated on interpretability and graph-based models. He is particularly excited about recent advances in applying abstract algebra, topology, and category theory to neural network design.
.jpg)
Cherie Xu
Senior Machine Learning Engineer
Team Members
ML Research Fellows
Since Arthur’s inception, our Research Fellows program has recruited and curated relationships with top AI, ML, policy, and legal junior researchers, who spend a summer or semester with Arthur building toward a joint goal of public dissemination of a research result. If you are a strong junior researcher interested in shaping the trustworthy and performant AI space, get in touch!
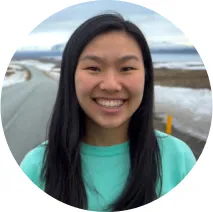
Angelina Wang
Angelina is a PhD student in Computer Science at Princeton University. She works on machine learning fairness and algorithmic bias, and is supported by the NSF GRFP. Previously she received her B.S. from UC Berkeley.
.webp)
Namrata Mukhija
Namrata is currently an Applied Scientist in the Machine Learning Center of Excellence team at JPMorgan Chase and Co. and holds an M.S. in Computer Science from New York University. Her research has previously involved building language technology for social good and developing interdisciplinary methods for the inclusion of marginalized communities in NLP. In addition to her time at Arthur, she has previously done research under Microsoft Research and JPMorgan Chase and Co. Her current research interests are in human-centered NLP and fairness in NLP.
.webp)
Michelle Bao
Michelle conducts research on interdisciplinary theory on AI ethics and practical tools for fairness, and hopes to better understand how one might inform the other. In addition to her time at Arthur, she has enjoyed doing research under various organizations including Stanford NLP Group, the ACLU, and Stanford ML Group and teaching/designing curricula for CS classes at Stanford.
.webp)
Naveen Durvasula
Naveen is an undergraduate at UC Berkeley. His research interests lie broadly at the intersection of theoretical computer science, machine learning, and economics. In particular, he's excited about applications of learning to mechanism design and new economic paradigms for data exchange. Naveen has worked on projects with applications in kidney exchange, ecommerce, matching theory, theoretical statistics, fairness, and machine learning operations. He has collaborated with researchers at the University of Maryland, UC Berkeley, and Harvard University.
.webp)
Lizzie Kumar
Lizzie Kumar is a Ph.D. candidate in Computer Science at Brown University. Her research analyzes computational and regulatory strategies for evaluating machine learning models from an interdisciplinary perspective. Previously, she developed actuarial risk models on the Data Science team at MassMutual. Lizzie holds an M.S. in Computer Science from the University of Massachusetts at Amherst and a B.A. in Mathematics from Scripps College.
.webp)
Kweku Kwegyir-Aggrey
Kweku is broadly interested in machine learning and statistics with a specific focus on the design of algorithms that audit machine learning models for fairness and robustness. He is interested in questions which rigorously examine and critique data-driven technological solutionism. He is a PhD candidate in the Brown University Department of Computer Science and received his bachelor’s degree in Computer Science & Mathematics at the University of Maryland.
.webp)
Sahil Verma
Sahil is a PhD student in the Department of Computer Science and Engineering at the University of Washington, Seattle. He is interested in answering questions related to explainability and fairness in ML models. In the past, Sahil has worked on developing novel techniques to generate counterfactual explanations for ML classifiers and also spearheaded a team that wrote a large and comprehensive survey paper on counterfactual explanations. Currently, Sahil is interested in problems of explainability in recommender systems and fairness in LLMs.