With the biggest commitment to mitigating climate change in U.S. history having recently been signed into law, climate is—even more so than usual—top of mind for many. From AI-based forecasts around extreme weather and CO₂ emissions to identification of climate-vulnerable regions, AI and machine learning technologies have been touted as the ultimate solution or silver bullet to overcoming climate change. Unsurprisingly, it’s not quite that simple.
Keep reading to find some use cases for AI and climate change, as well as the ways in which AI is simultaneously solving and contributing to the phenomenon.
The Pros
In so many ways, these technologies can be absolute game-changers when it comes to mitigating the effects of climate change. In fact, 87% of private and public sector CEOs with decision-making power in AI and climate believe AI is an essential tool in the fight against climate change. Below are just a few of the more common use cases.
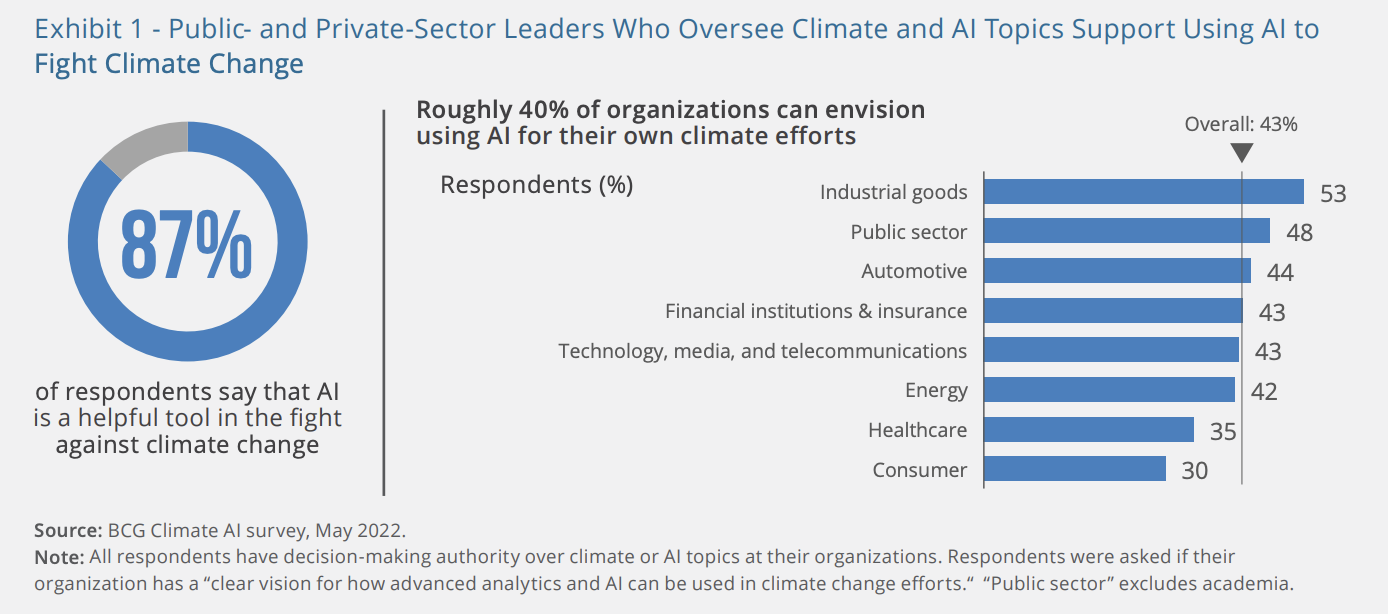
Mitigation
This is one of the most crucial use cases for AI: the measurement, reduction, and removal of emissions and GHG effects.
- Measurement: Measuring emissions, both in the overall environment and at the level of individual products and actions, allows us to take stock of our current situation and forecast future trends. It also allows us to prioritize the abatement efforts with the highest potential to reduce emissions, at both the macro level and the micro level.
- Reduction: AI solutions can reduce emissions intensity by improving supply forecasts for intermittent renewable energy sources or creating more efficient electric-grid balancing. Additionally, they can reduce emissions-generating activities by optimizing supply chains, such as through improved demand forecasting or more efficient transportation of goods.
- Removal: AI-based solutions can be used to support and more accurately quantify the environmental removal process by analyzing satellite images to detect deforestation and estimate ecosystem carbon sequestration. They can also be used for technological removal efforts such as direct air capture (DAC) or carbon capture by assessing capture and storage locations, monitoring leakage, and optimizing the industrial processes and materials used for carbon capture.
Adaptation & Resilience
Adapting to climate change is a critical undertaking for policymakers and the public, as it boosts resilience to the effects of long-term climate trends and extreme events. Solutions can apply AI to promote adaptation and resilience, particularly in hazard forecasting and in vulnerability and risk-exposure management.
- Hazard Forecasting: AI is well suited to help in forecasting and projecting climate-related hazards, whether in the form of improved long-term projections of regionalized events, such as sea-level rise, or in the form of early warning systems for extreme weather events such as hurricanes or droughts.
- Vulnerability & Risk-Exposure Management: AI can be used here to enable public- and private-sector leaders to make more informed decisions during crises, strengthen infrastructure through predictive maintenance of structures, protect populations through the prediction and monitoring of things like large-scale migration patterns or food insecurity risk, and preserve biodiversity through systems like intelligent irrigation.
Fundamentals
AI can be used to support research and education efforts on climate change, helping stakeholders understand the risks and implications and share what they learn. All such efforts reinforce and magnify progress toward mitigation and toward adaptation and resilience. AI can be used to bolster climate research and modeling; climate finance and analytics; and education, nudging, and behavior change (such as in the form of recommendations for environmentally friendly purchases).

The Cons
On the other hand, the carbon footprint and computational costs of training large machine learning models can’t and shouldn’t be overlooked.
In a recent publication, researchers from the University of Massachusetts at Amherst conducted a life cycle analysis for training several typical big AI models. They discovered that the process may produce over 626,000 pounds of CO₂ equivalent—which is 5x more than a U.S. car produces in its entire lifetime and a whopping 57x more than one human produces in a year.
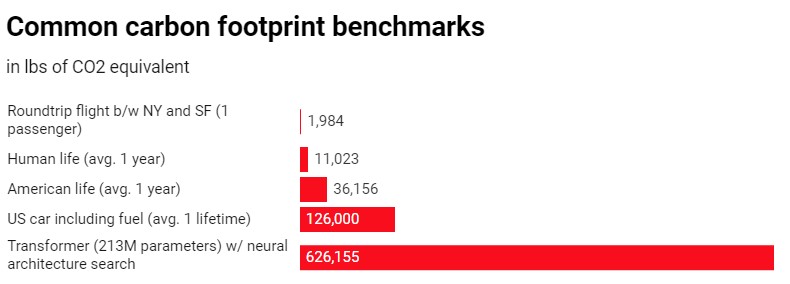
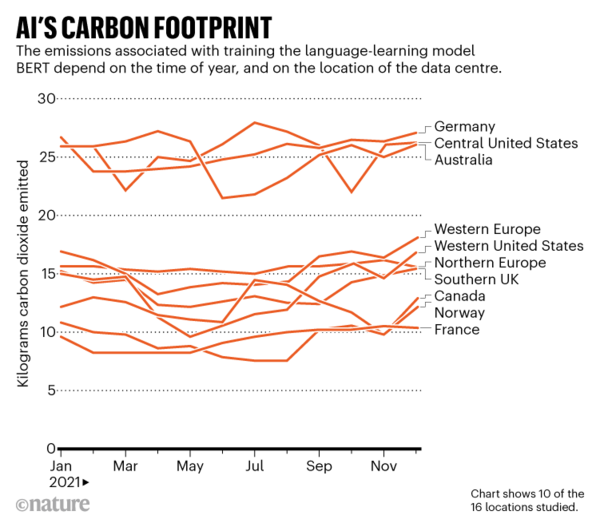
Artificial intelligence algorithms, which power some of technology’s most cutting-edge applications, such as producing logical stretches of text or creating visuals from descriptions, may need massive amounts of computational power to train—and their energy demands are rapidly increasing. The computing resources required to create a best-in-class AI model have doubled every 3 to 4 months in the deep learning era. This, in turn, necessitates a vast quantity of power, prompting many to fear that the carbon footprint of these increasingly popular ultra-large AI systems will render them environmentally unsustainable.
Here are a few ways that the carbon footprint of AI can be reduced:
- Make use of computationally efficient machine learning algorithms.
- Don’t train a model from scratch if it is not necessary.
- When you can, use Automated ML.
- Since the model inference accounts for 80-90% of the model cost, use customized processors that increase the speed and efficiency of training and testing neural networks.
AI is a tool that is already helping us and will continue to help us build a more resilient future—but, like all tools, it should be used wisely. Additionally, solving the climate change crisis requires not just technological innovation, but also the will of decision makers to take action and make the necessary changes toward a better future.
Interested in learning how Arthur can help your organization monitor, measure, and improve AI efforts? Schedule a demo.