A 2021 365 Data Science Ltd study revealed that data science professionals remain with an employer for only 1.7 years on average. In fact, the sudden impulse to write a data science resignation letter doesn’t change depending on where you work in the world. The study data sampled data science professionals from the US (35%), the UK (25%), the EU (25%), and India (15%).
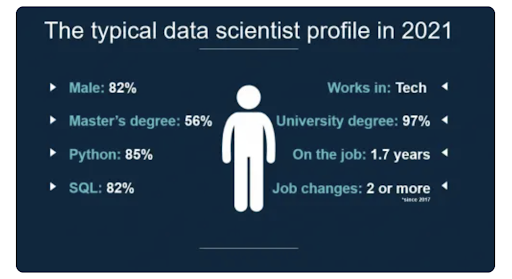
So, when it comes to maintaining talent, what can employers do to combat data scientists’ desire to change jobs and keep them fulfilled in their roles?
#1 Pay competitive salaries and incentivize with annual bonuses and equity refreshes.
Currently, demand outstrips supply. It’s simple economics. According to the U.S. Bureau of Labor Statistics, the expected job market growth rate for data science and related fields is 31%, far greater than the overall average job growth rate of 4%. When there are more jobs than candidates, data scientists can easily jump jobs and get a pay bump because they have more salary bargaining power.
#2 Carve out and create dedicated weekly innovation project time for team members.
In a recent Medium blog post authored by Ian Xao, he noted that “data science teams at big companies are facing a serious retention problem” as scientists are quitting enterprise companies for new jobs at startups.

Xao pointed out that many data scientists have an expectation-reality gap, leading to day-to-day job disillusion. Data scientists desire to work with advanced algorithms and troubleshoot models—but they end up spending up to 40% of their time on data collection, data cleaning, and tedious administrative manual data export-import activities to keep up with reporting demands.
Data scientist Natassha Selvaraj echoed Xao’s sentiment on a recent KDNuggets post:
“At the end of the day, you’re no longer building complex algorithms and predictive models like you imagined. You now spend all your time brushing up on SQL and data preparation skills to pull data out of the system into different formats, and present this data to stakeholders so they can use it to make business decisions. Although your job title has the word ‘data science’ in it, you aren’t in a role you’ve always pictured yourself in. You’re unhappy being the company’s data janitor, and want to work on projects that will actually utilize the skills you’ve spent so long to gain.”
Giving data scientists autonomy to work on model innovation or experimentation (i.e. passion projects) for a set percentage of time (10% to 20%) each week keeps your employees engaged and empowered because they feel a sense of ownership and control over their role.
#3 Automate documentation workflows and reporting activities to reduce admin burdens.
Documentation, documentation, documentation (cue Jaws theme). As AI/ML regulation matures and places greater pressure on higher up model risk managers to document rationale and explainability behind data sets and model decision making, repetitive reporting asks often trickle down to data scientists and become a dreaded chore. This can range from design docs to writing model cards for communicating to external or non-technical stakeholders deployed model context, performance evaluation criteria and other relevant information. Or it could be a more in-depth report to satisfy an DPIA (data protection impact assessment), internal, or third-party audit requirement.
Investigate MLOps platforms that can automate a team’s manual, repeatable activities. By automating univariate and multivariate data drift detection, event logging, data exporting for monthly/quarterly reporting requirements and more, your team can break the chains from day-in, day-out data science “janitorial” maintenance and focus on complex model diagnosis and mitigation solutions.